– My name is Dennis Fremstad. I am from Røros, Norway.
Dennis is an old acquaintance at the Institute of Theoretical Astrophysics (ITA): he got both his Bachelor's and Master's at the University of Oslo (UiO) through the physics and astronomy bachelor programme and the astronomy master programme. In August he started working as Ph.D student, moving from the basement up to the fifth floor of the building.
– Before I started my Ph.D I did a master in astrophysics at ITA, on the topic adapting machine learning into astrophysics. During this project I made an emulator that can make predictions of the non-linear matter power-spectrum with mixed Axion dark matter.
Adapting machine learning into cosmology
– For my Ph.D I am continuing working on the role of machine learning in cosmology, with a project focused on enhancing the resolution of simulations and exploring other applications of this approach, begins Dennis.
– What's the main question of your research?
– The main focus of the project is to explore how we can use Artificial Intelligence (AI) to assist in cosmology. One way that this has been done is by using machine learning to enhance the resolution of pure dark matter simulations, which avoids the need to run large, high resolution simulations, begins Dennis.
– What we would like to do, is to investigate if we can use this algorithm to create mappings between low resolution pure dark matter simulations and other fields, such as the Axion scalar field, gas or dark matter fields using models of gravity different from Newtonian, he continues.
"This way, we can add new physics to the simulations through machine learning".
– What is your approach?
– There are two main parts of this project; the data and the algorithm.
The algorithm that Dennis would like to use is known as a Generative Adversarial Network (GAN). This consists of a generator (which tries to replicate the target data, essentially trying to make new data and pass it as real) and one discriminator (which tries to discern real data from fake data). As these networks improve, the generator gets better at replicating the target data and the discriminator gets better at discovering if the data is real or fake.
– The end goal is to create a generator which understands the underlying statistics of the target data and thus can create new, authentic data without simply creating a copy of the target data, he explains.
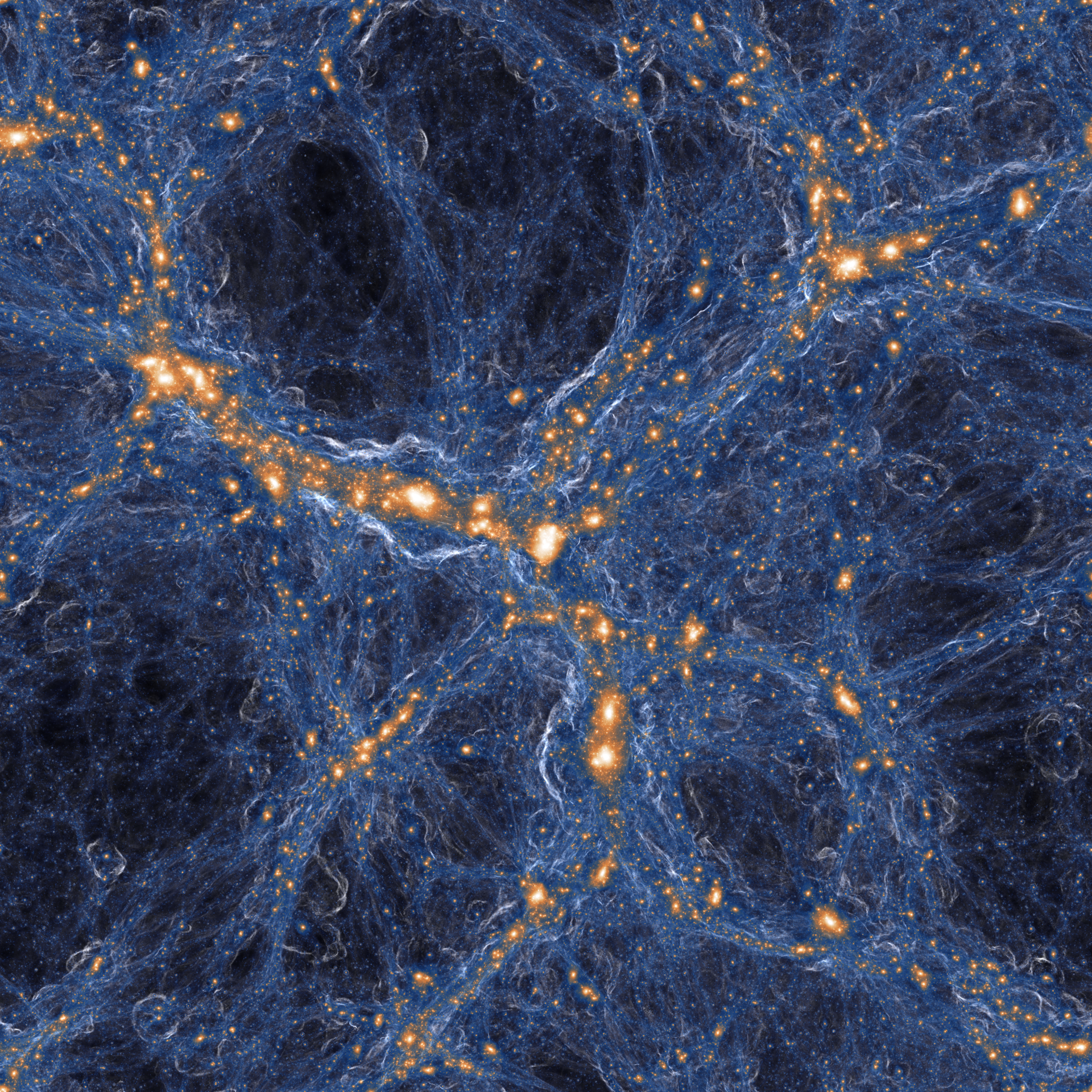
– To train this network we require several low resolution dark matter simulation boxes as well as their high resolution counterpart. This means that we either have to run simulations (using e.g. gevolution, axioNyx or TNG) or use publicly available data with sufficiently high resolution (like data from the illustrisTNG project).
Machine learning for answering the big questions
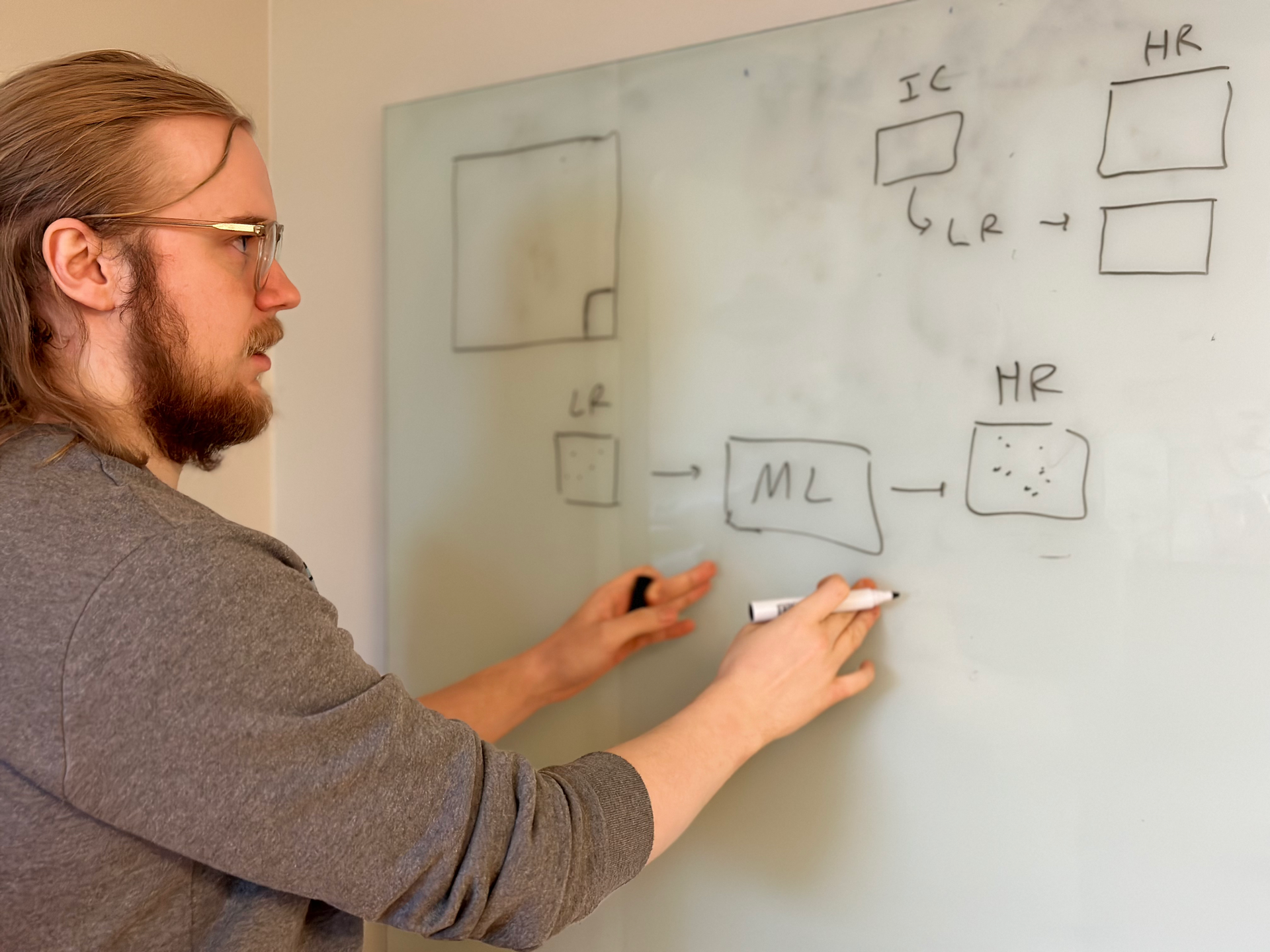
– What fascinates you about your research?
– I am most fascinated about the use of machine learning, how it can be applied to different fields and what applications it can have for society in the future, tells Dennis.
"What is the origin of the universe and what rules governs it? Perhaps the use of machine learning could help us take a step towards answering these questions".
Working with the brightest minds in the field
– What brought you to Oslo?
– I was drawn to study in Oslo with a hope of better weather. As someone who has walked to school in temperatures down to -35 degrees, I was ready for something warmer. Studying a bachelor in physics and astrophysics, at one point we got to choose between which path we wanted to pursue. Since I had more fun, and thought it was much more interesting, I chose to pursue astrophysics. Wanting to stay in Oslo, I chose to study my masters at ITA and since I enjoyed my time there I chose to pursue a Ph.D here.
– What is your experience at ITA so far?
– My experience here at ITA and in Oslo has been incredible! I have had the opportunity to engage in many different projects and collaborate with some of the brightest minds in the field.