About the project
Glaciers are under pressure in the current climate warming trend. The aerial extent and the mass balance, a measure of mass loss or mass gain of a glacier are representative of the “health state” of a glacier. They are recognized as Essential Climate Variables by the World Meteorological Organization. Due to the sheer number of glaciers of roughly 200.000 worldwide and their inaccessibility, satellite data has been proven as a valuable source to map glaciers around the globe.
Satellite imagery has enabled researchers to map the extent of glaciers and glacier surface types (glacier facies, i.e. snow, firn, ice). Multi-temporal information on the glacier surface elevation and mass balance proxies (snow cover, albedo) which are highly correlated with the glacier mass balance have been used to estimate mass changes. Today the ever-growing amount of satellite data motivates the development of new methodology to maximize the information retrieved (value-added) and ease the handling and storage of the data in data cubes.
Recently, the field of machine learning has been revolutionized by deep learning. In the context of image analysis, Convolutional Neural Networks are among the most successful deep learning algorithms as they can learn a hierarchy of spatial features at different layers of the network associated to increasing levels of abstraction, i.e., from raw pixel values to parts of objects (edges and corners), local shapes, up to complex structures in satellite and aerial images.
In MASSIVE the most promising network architectures for pixel-wise mapping in remote sensing, Fully Convolutional Networks, will be adapted to map glacier extend and estimate glacier mass balance.
Objectives
In MASSIVE, the project team aims at improving glacier mapping and surface glacier mass balance estimation techniques with the help of machine learning, especially deep learning. We will develop the methodology for glaciers in Norway, Svalbard, the European Alps and the Himalayas and then expand it to regions with different glacier characteristics.
The project outcome will be a multi-temporal glacier inventory and a multi-annual time series of mass balance of the glaciers under investigation. This data can support national authorities to report on glacier mass balance, be used as input for hydrological run-off modelling and global glacier mass balance models. The data cubes of glacierized regions will be open-access to foster future research on these sensitive climate indicators.
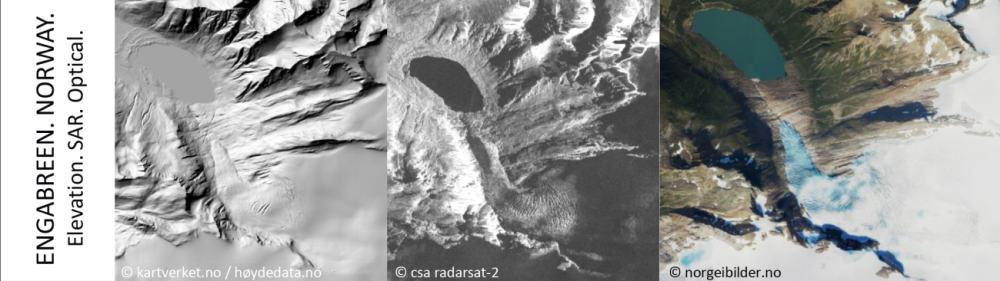
Financing
This research project is funded by the “Forskerprosjekt for fornyelse-programme” of The Research Council of Norway. The project number at NFR is 315971.
The MASSIVE research project is funded over the 4 year period, from 2021 to 2025.
Collaboration
The MASSIVE-project is a collaboration from researchers in Norway, Italy and The Netherlands. The partners are:
- Department of Geosciences, University of Oslo, Norway
- Institute for Earth Observation EURAC, Italy
- Faculty of Geo-Information Science and Earth Observation (ITC), University of Twente, Netherlands
- The Norwegian Water Resources and Energy Directorate (NVE), Norway
- Statkraft, Norway