Prøveforelesning - tid og sted
Fredag 25 oktober, 10:15-11:00, Auditorium 1, Geologibygningen:
The potential and limits of modeling snowmelt, ice melt, and rainfall-runoff in the subarctic region
Kreeringssammendrag
Modellering av vannstrømmer og snømengder kan være utfordrende på grunn av mange kilder til usikkerhet. Avhandlingen har fokus på usikkerhetskvantifisering og reduksjon i hydrologiske modelleringer gjennom bruk av både eksisterende og nylig introduserte metoder. Et av de viktigste resultatene av arbeidet var å adoptere et eksisterende rammeverk for usikkerhetsanalyse for å få det nyttige ut av de ufullkomne modelleringene i typiske driftsmodeller. Det ble også vurdert muligheten til å anvende tre maskinlæringsmodeller for å minimere beregningstid ved bruk av denne tilnærmingen. Et annet hovedresultat var å forbedre to data assimileringsmetoder i forhold til deres evne til å trekke ut tilgjengelig informasjon fra et datasett med mange feilaktige verdier, og slik redusere usikkerhet i en prediksjon..
Hovedfunn
Populærvitenskapelig artikkel om Teweldebrhans avhandling:
Although hard to avoid, we can deal with modelling uncertainty
In the contemporary world, many environmental and water resources related decisions rely upon wide range of modelling results. However, models are simplified representations of reality and their predictions are generally imperfect due to the inherent uncertainties (errors) emanating from various sources. Therefore, there is an increasing demand to incorporate uncertainty analysis as part of the modelling process. This PhD thesis was, thus, focused on uncertainty quantification and reduction in hydrological modelling through use of both existing and newly introduced methodologies.
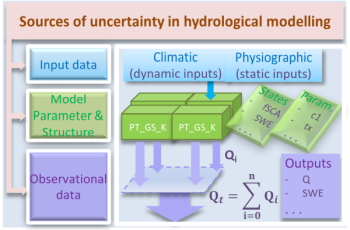
The viability of these methodologies was evaluated using the Statkraft’s hydrological model and relevant climatic and physiographic datasets from the Nea-river catchment in Trøndelag county, in Mid-Norway.
One of the major outcomes of the PhD work was the adoption of an existing rejectionist uncertainty analysis framework as a ‘tool’ to find the useful out of the imperfect models. Further, machine learning models were coupled with the adopted uncertainty analysis framework in order to minimize the computational time when using this approach. Another major achievement was the improvement in the efficiency of two data assimilation schemes in extracting the available information from a ‘noisy’ dataset and thus reducing the prediction uncertainty. The research outputs from this work will aid water managers in quantifying and reducing modelling uncertainty and thereby in making rational decisions with regards to use of the valuable, but often scarce, water resources.
Foto og annen informasjon:
Pressefoto: Aynom Teweldebrhan (portrett)
Annet bildemateriale: Diagram over main sources of uncertainty which are present in hydrological modelling and predictions. Graphics/600px: Aynom Teweldebrhan. | Physiography and location map of the Nea-river catchment in Norway. Graphics/600px. Aynom Teweldebrhan